Researchers frequently employ the use of a maze to observe the learning capabilities of mice and rats. With the development of increasingly sophisticated robots, scientists are curious if these devices can also navigate such a puzzle. A team at the Eindhoven University of Technology (TU/e) in the Netherlands and the Max Planck Institute for Polymer Research in Mainz, Germany have completed a study that demonstrates they can—by mimicking the human brain.
The study also lays the groundwork for innovative new ways to apply neuromorphic devices in the areas of health and more. Recent years have seen a considerable increase in the popularity of machine learning and neural networks, especially considering their efficacy in areas like image recognition, medical diagnosis, e-commerce and many others. Nevertheless, there are downsides to this technology, most notably its energy inefficiency and high power consumption.
Addressing this power problem is a main motivator for researchers working on the development of energy efficient computers. Many look for answers in the human brain, which is highly efficient due to its combined memory and processing. The neurons in the brain use synapses to communicate with each other, and these synapses grow stronger and stronger as more information flows through them. This enables human beings to learn and remember everything from stories to faces.
“In our research, we have taken this model to develop a robot that is able to learn to move through a labyrinth,” says Imke Krauhausen, principle author and PhD student at the department of Mechanical Engineering at TU/e, in a statement. “Just as a synapse in a mouse brain is strengthened each time it takes the correct turn in a psychologist’s maze, our device is ‘tuned’ by applying a certain amount of electricity.”
Lego robot behind breakthrough
Researchers used the Mindstorms EV3 robot from a robotics kit made by Lego. Mindstorms EV3 has two wheels, traditional guiding software enabling it to follow a line, and many reflectance and touch sensors. These technologies made researchers confident it would be able to navigate the honeycomb-like maze made of black-lined hexagons they had built for it.
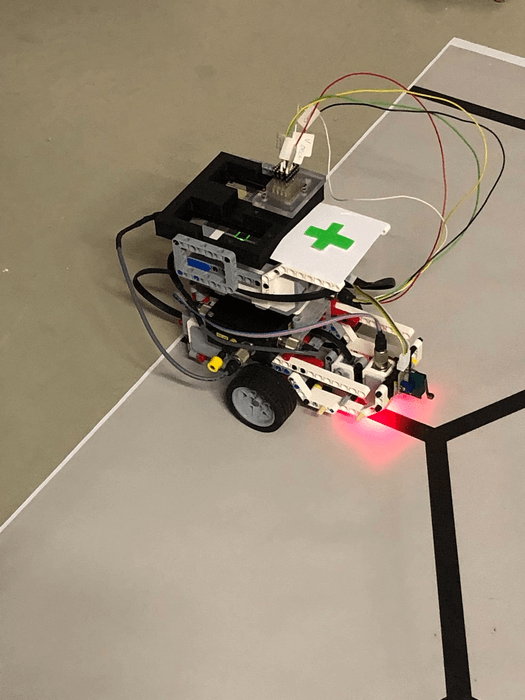
The maze had a designated path leading to the exit indicated by visual clues. The robot navigated the maze using its programmed default right turns until it reached a dead end or diverged from the path to the exit. When it made such an error, it would either turn left or return to its previous position. The robot then remembers the corrective stimulus in the neuromorphic device as it continues to make its way through the maze.
“In the end, it took our robot 16 runs to find the exit successfully,” Krauhausen says. “And, what’s more, once it has learned to navigate this specific route, it can navigate any other path that it is given in one go. So, the knowledge it has acquired is generalizable.”
The robot is able to navigate its way through the maze in part thanks to the sensors and motors carefully integrated into its makeup. “This sensorimotor integration, in which sense and movement reinforce one another, is also very much how nature operates, so this is what we tried to emulate in our robot,” said Krauhausen.
Another unique feature of the robot is its ability to remember its mistakes and corrections and apply its learnings to future challenges. This was achieved thanks to the polymer organic materials used to make the neuromorphic robotic device. The polymer known as p(g2T-TT)) is both stable and able to ‘retain’ much of its learned behavior, similarly to how neurons and synapses in human brains remember actions or events.
‘Where devices are going in the future’
Using polymer instead of silicone in the development of neuromorphic devices is a very new method first introduced by co-authors of this paper Paschalis Gkoupidenis of the Max Planck Institute for Polymer Research in Mainz and Yoeri van de Burgt of TU/e. Their research was able to demonstrate that polymer organic materials are able to be tuned across a much larger range of conduction than its inorganic counterparts, in addition to its improved “memory” of learned states for extended periods of time.
Now, the use of organic materials in these devices has become increasingly popular in the field.
“Because of their organic nature, these smart devices can in principle be integrated with actual nerve cells,” says Krauhausen. “Say you lost your arm during an injury. Then you could potentially use these devices to link your body to a bionic hand.”
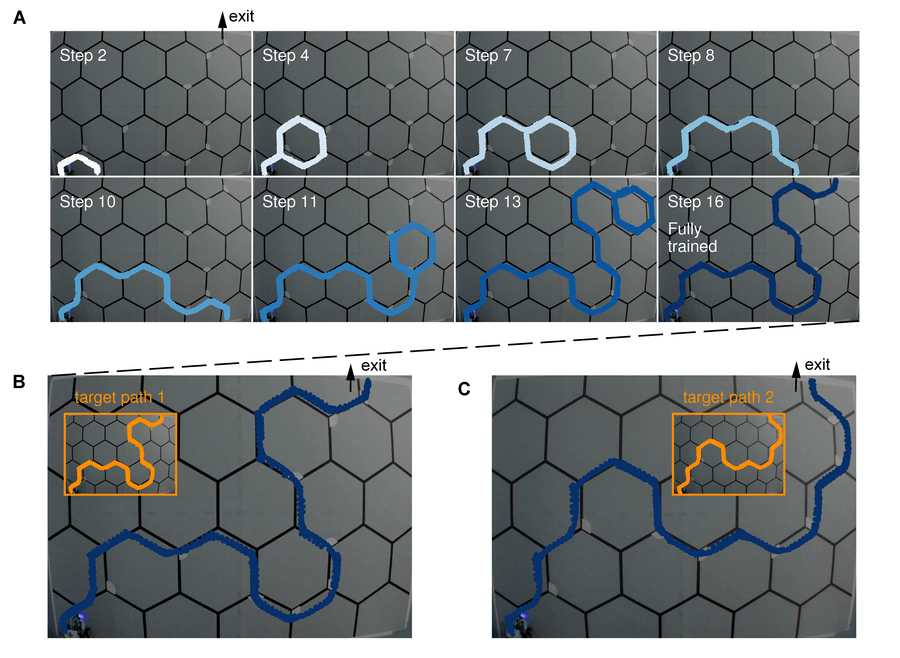
Organic neuromorphic computing may also be usefully applied in small edge computing devices, which processes data from sensors locally and outside of the cloud.
“This is where I see our devices going in the future,” said van de Burgt. “Our materials will be very useful because they are easy to tune, use much less power, and are cheap to make.”
Researchers now wonder if neuromorphic robots will be able to one day play in a game of soccer as TU/e’s soccer robots have already done successfully.
“In principle, that is certainly possible, but there’s a long way to go. Our robots still rely partly on traditional software to move around,” Krauhausen says. “And for the neuromorphic robots to execute really complex tasks, we need to build neuromorphic networks in which many devices work together in a grid. That’s something that I will be working on in the next phase of my PhD research.”
This study is published in Science Advances.
Article written by Anna Landry